Yamir Moreno
Multilayer systems: from diffusion processes to transportation networks
Multilayer networks are a new paradigm in the study of complex interconnected systems, as they allow to cast into a single framework the complex topological and dynamical interdependencies between different networked systems. In this talk, we first provide a brief overview of what is the state-of-the-art in network science, paying special attention to multilayer networks. Then, in order to discuss some specific case studies, we present results concerning how to characterize diffusion processes that take place on top of these systems as well as on what new insights can be gained using multilayer approaches to study interdependent transportation systems.
​
Sanjukta Bhowmick
How Network Structure Affects its Stability
Networks created from real-world data inevitably contains some noise, which is manifested as small changes in the network structure. Often, due to this change in structure, the ranking of the high centrality vertices also alters. We define a network as stable if the change in ranking is not very high. In this talk, I will discuss which structural properties of the network affect the stability and bounds on how much the ranking can change.
Sayan Pathak
Unstructured text/language understanding using Deep Neural Nets
Deep Neural Networks (DNNs) have revolutionized the way that machines understand language and have allowed us to create models that answer textual questions, translate pairs of languages, and intelligently compare document corpora. At the heart of these successes lie core techniques that fall into the area of deep NLP and sequence to sequence parsing. While powerful, dealing with variable-size sequences in DNNs requires deep understanding and experience in creating such networks, which can be daunting to many scientists and engineers. Microsoft Cognitive toolkit (formerly CNTK) provides easy to use recipes to perform these tasks that scales to very large text corpus. In this talk, I will introduce different features of the toolkit that enable such scalability. I will show walk through two recipes one on text classification and another sequence to sequence translation that can be applied to a variety of machine learning tasks.
​
Daniel Larremore
Dynamics of Beneficial Epidemics
Pathogens can spread epidemically through populations. Beneficial contagions, such as viruses that enhance host survival or technological innovations that improve quality of life, also have the potential to spread epidemically. How do the dynamics of beneficial biological and social epidemics differ from those of detrimental epidemics? We investigate this question using two theoretical approaches, which combine, on the same timescale, dynamics on a network and dynamics of the network. In the first approach, in the context of behavioral epidemiology, we show that infections that cause increased connectivity lead to superexponential fixation in the population. In the second approach, in the context of dynamic social networks, we find that preferences for increased global infection accelerate spread and produce superexponential fixation, but preferences for local assortativity halt epidemics by disconnecting the infected from the susceptible. Generally, the dynamics of beneficial epidemics are fundamentally different than the dynamics of detrimental epidemics, and leave traces of their spread in the structure of the resulting reconfigured networks.
Yérali Gandica
Communities-based Networks as a Consequence of homophily effects
Understanding the emergence of strong controversial issues in modern societies is a key issue in opinion studies. A commonly diffused idea is the fact that the increasing of homophily in social networks, due to the modern ICT, can be a driving force for opinion polarization. In this talk, I will address the problem with a modelling approach. I will show how we first introduce a network morphogenesis model, where homophily can be tuned with a parameter. We show that as homophily increases the emergence of marked topological community structures in the networks raises. Secondly, we perform an opinion dynamics process on homophily dependent networks and we show that, contrary to the common idea, homophily helps consensus formation. Finally, the modelling hypotheses are tested in several datasets.
Márton Pósfai
Controllability of temporal networks and multiplex, multi-timescale networks
Structural controllability exploits the deep connection between graph combinatorics and linear algebra, making it possible to answer control related questions relying on network structure only. Recent work demonstrated that the underlying networks of complex systems alone is a source of non-trivial control phenomena. However, in real complex systems there are other important sources of complexity. In my talk I will demonstrate how to extend structural controllability to take into account two such scenarios: temporal networks, where the timescale of dynamics of the network is comparable to the timescale of the dynamics on the network; and multiplex, multi-timescale networks, where the timescale of the dynamics of each layer of interaction is different.
​
Michael Schaub
Spectral techniques for network learning
Spectral algorithms have been shown to be powerful tools for a variety of tasks in network science. In this talk we will review how the spectral properties of the graph Laplacian and related algebraic representations are related to the structural features of a network, focusing in particular on (almost) invariant subspaces induced by the network structure. We discuss how these insights can be used in three application scenarios: 1) the analysis of nonlinear oscillator networks, ii) the inference of (hierarchical) stochastic block-models from network data, and iii) the inference of the exact network structure based on observing a set of measurements describing the state vectors of a consensus dynamics in response to Gaussian inputs.
Alexey Medvedev
Modelling the dynamics of discussion threads with temporal Hawkes branching processes: the case study of Reddit
Internet message boards provide a platform for social discussion of news and events that happen around us every day. On these boards, people stand as creators of such discussions, by starting a thread on a specific matter that they usually are concerned about, which further attracts attention of other people, who become participants in those discussions. The trace left by the discussion is the ‘discussion tree’, in which nodes represent post and comments, and an edge represents a ‘reply-to’ relation. Discussion trees have already gained attention of many scientists, who studied various static properties of them. In the following talk, we study the dynamics of discussion trees’ generation, based on the dataset of all Reddit threads from Jan, 2007 to May, 2017. We disregard the content of posts and comments and analyse only temporal information. We find universality in the arrival time distribution of comments to posts, as well as comments to comments, and show the power-law decrease of attention to threads within the first day of the post’s lifetime. We propose a dynamic temporal model based on Hawkes branching processes, that well recreates the structure and the dynamics of the discussion threads, thus claiming that its structure is close to random. The proposed model can be also used for prediction of some properties of discussion trees.
Lucas Jeub
A Local Perspective on Community Structure in Multilayer Networks
The analysis of multilayer networks is among the most active areas of network science, and there are now several methods to detect dense “communities” of nodes in multilayer networks. One way to define a community is as a set of nodes that trap a diffusion-like dynamical process (usually a random walk) for a long time. In this view, communities are sets of nodes that create bottlenecks to the spreading of a dynamical process on a network. We analyze the local behavior of different random walks on multiplex networks (which are multilayer networks in which different layers correspond to different types of edges) and show that they have very different bottlenecks that hence correspond to rather different notions of what it means for a set of nodes to be a good community. This has direct implications for the behavior of community-detection methods that are based on these random walks.
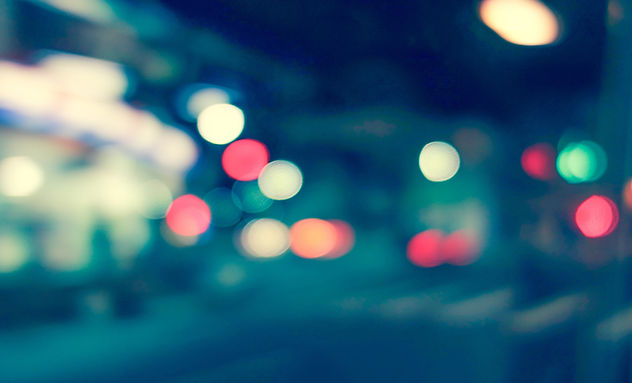
Titles and Abstracts
Michael Schaub
Spectral techniques for network learning
Spectral algorithms have been shown to be powerful tools for a variety of tasks in network science. In this talk we will review how the spectral properties of the graph Laplacian and related algebraic representations are related to the structural features of a network, focusing in particular on (almost) invariant subspaces induced by the network structure. We discuss how these insights can be used in three application scenarios: 1) the analysis of nonlinear oscillator networks, ii) the inference of (hierarchical) stochastic block-models from network data, and iii) the inference of the exact network structure based on observing a set of measurements describing the state vectors of a consensus dynamics in response to Gaussian inputs.