
Katharina Anna Zweig
On the degree centrality in multiplex networks - The paper that kills complex network analysis
In almost any network analytic project, we make multiple, innocent, and seemingly inconsequential modeling decisions - however, some of them might have a profound impact on the results.
In this talk, I will discuss how to evaluate the most simple centrality index, the degree, of a node in multiplex networks. Of course, any ranking of all nodes in a multiplex network requires first, some normalization of the degrees to put them on the same scale, and second, some aggregation over the different layers. You might opt for the sum, the average, the mean, the minimum or the maximum as an aggregation strategy. In this talk I will show that the combination of these two strategies, i.e., normalization and aggregation, may result in nodes being identified as either top central or least central. It thus emphasizes the need to properly describe, check, and evaluate even the most simple modeling decisions.
Markus Strohmaier
Understanding Markovian Network Dynamics
In this talk I will talk about approaches for understanding transition behavior on networks. In particular I will first introduce HypTrails - a Bayesian framework for comparing different hypotheses
about human transition behavior in networks. Then I will introduce SubTrails - an approach for identifying subgroups of users with exceptional transition behaviors. I will conclude with open challenges
and problems in the field.
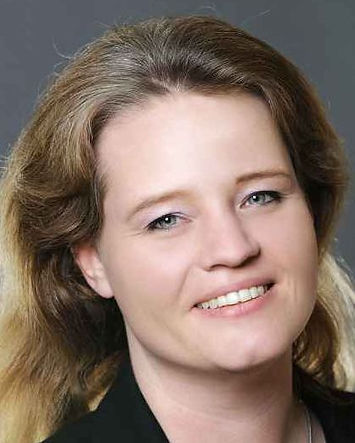
Tiago P. Peixoto
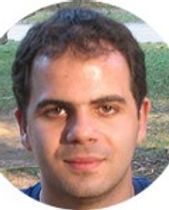
Krishna P. Gummadi
Discrimination in Human vs. Algorithmic Decision Making
Algorithmic (data-driven) decision making is increasingly being used to
assist or replace human decision making in a variety of domains ranging
from banking (rating user credit) and recruiting (ranking applicants) to
judiciary (profiling criminals) and journalism (recommending
news-stories). Against this background, in this talk, I will pose and
attempt to answer the following high-level questions:
(a) Can algorithmic decision making be discriminatory?
(b) Can we detect discrimination in decision making?
(c) Can we control algorithmic discrimination? i.e., can we make algorithmic decision more fair?

Julien Siebert
Understanding fashion as a complex network
Fashion is a very fast moving business addressing many different target groups (style, age, occasion, status, …). Hence, it is hard to predict what will be fashionable in the future. Originally, how these markets evolved was largely dictated by a relatively small set of players, such as designers, brands or celebrities. Nowadays, social media is to some extend changing the rules of play. Bloggers and other participants in social media are increasingly playing an important role in defining and spreading fashion trends. Zalando wants to take active part in this development. Some of our goals are to discover new influencers in the fashion world, match influencers to specific brands or advertising campaigns and to discover and to monitor emerging trends present in social media. To do this, we have to construct a large network of entities (such as bloggers, brands, magazines) to be able to analyze the dynamic behavior of the fashion world and answer the questions mentioned above. For this talk, we studied a very popular fashion platform with around 1M subscribers and 22M connections. We will address questions such as which geographical regions of the world are most active or if people tend to follow influential users from their same country or region. In addition, we will analyze if some of the standard properties of complex networks apply in our example, such as small world, scale free, etc.

Matthieu Latapy
Stream Graphs and Link Streams for the Modeling of Interactions Over Time
The structure and dynamics of interactions is crucial for many phenomena of interest, like contacts between individuals, data transfers, commercial exchanges, mobility, and many others. Analyzing such interactions classicaly relies on network analysis, which captures the structure of interactions, or on temporal series, which captures their dynamics. Both approaches have been extended in various ways to cope with the both structural and temporal nature of interactions, but current situation remains unsatisfactory. I will present here the modeling of interactions over time by stream graphs and link streams, which aims at unifying both aspects into a simple, efficient and intuitive way. It provides a language to deal with interactions over time, in a way
similar to the language provided by network science for relations.
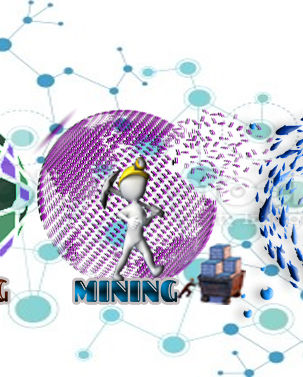
Modeling sequences and temporal networks with dynamic community structures
Community-detection methods that describe large-scale patterns in the dynamics on and of networks suffer from effects of arbitrary time scales that need to be imposed a priori. We develop a variable-order hidden Markov chain model that generalizes the stochastic block model for discrete time-series as well as temporal networks. With our model, the relevant time scales are inferred from data, instead of being determined beforehand. The temporal model does not require the aggregation of events into discrete intervals, and instead takes full advantage of the time-ordering of the tokens or edges. When the edge ordering is random, we recover the traditional static block model as a special case. We formulate an efficient nonparametric Bayesian framework that can select the most appropriate Markov order and number of communities, based solely on statistical evidence and without overfitting.

Vito latora
Phase Transitions in the Growth of Spatial Networks?
Spatially embedded complex networks, such as nervous systems, the Internet, and transportation networks, generally have nontrivial topological patterns of connections combined with nearly minimal wiring costs. We report here the empirical analysis of two databases describing respectively: 200 years of evolution of the road network in a large area located north of Milan (Italy), and the growth of the nervous system of the C. elegans from the moment of fertilization to adulthood. We discuss the basic mechanisms that drive the evolution of such two spatial networks.

Renaud Lambiotte
Burstiness and spreading on networks: models and predictions
When modelling dynamical systems on networks, it is often assumed that the process is Markovian, that is future states depend only upon the present state and not on the sequence of events that preceded it. Examples include diffusion of ideas or diseases on social networks, or synchronisation of interacting dynamical units. In each case, the dynamics is governed by coupled differential equation, where the coupling is defined by the adjacency matrix of the underlying network. The main purpose of this talk is to challenge this Markovian picture. We will argue that non-Markovian models can provide a more realistic picture in the case of temporal networks where edges change in time, or in situations when pathways can be measured empirically. We will focus on the importance of non-Poisson temporal statistics, and show analytically the impact of burstiness on diffusive dynamics, before turning to applications and incorporating memory kernels in predictive models of retweet dynamics.
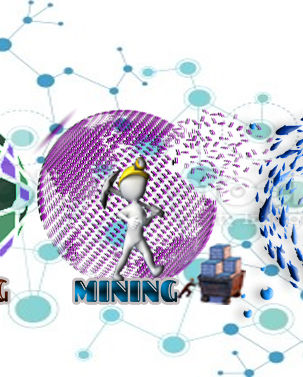
Eun Lee
The Effects of Correlation between Influential Level and Threshold in Opinion Dynamics
In every choice we make, repetitive interactions within our social networks and our susceptibility to influence impact our decisions. This research investigates such mechanisms with the framework of threshold models of social contagion. We find that a strong positive correlation between the degree (representing the strength of influence) and the threshold (displaying acceptance level) slows the dynamics and segregates the opinions.
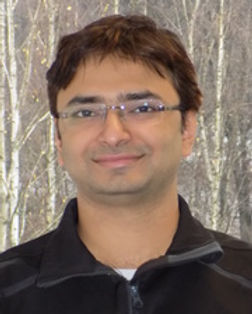
Rishiraj Saha Roy
Syntactic Complexity of Web Search Queries through the Lenses of Language Models, Networks and Users
Across the world, millions of users interact with search engines every day to satisfy their information needs. As the Web grows bigger over time, such information needs, manifested through user search queries, also become more complex. However, there has been no systematic study that quantifies the structural complexity of Web search queries. In this research, we make an attempt towards understanding and characterizing the syntactic complexity of search queries using a multi-pronged approach. We use traditional statistical language modeling techniques to quantify and compare the perplexity of queries with natural language (NL). We then use complex network analysis for a comparative analysis of the topological properties of queries issued by real Web users and those generated by statistical models. Finally, we conduct experiments to study whether search engine users are able to identify real queries, when presented along with model-generated ones. The three complementary studies show that the syntactic structure of Web queries is more complex than what n-grams can capture, but simpler than NL. Queries, thus, seem to represent an intermediate stage between syntactic and non-syntactic communication.
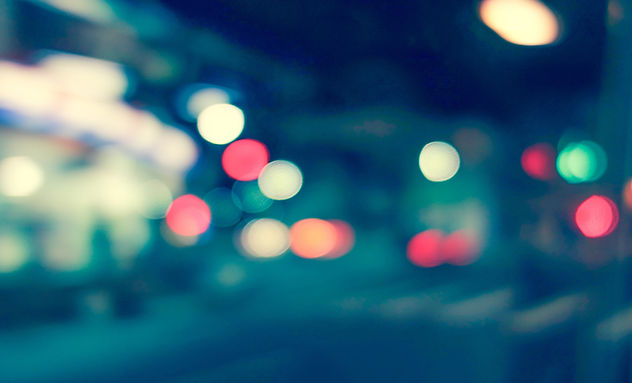
Titles and Abstracts
#DOOCN2016